Direct things from here in EXAM
Chemistry Formula Sheet
https://c360.me/a8e0e8
https://c360.me/a8e0e8
https://c360.me/a8e0e8
Ye karliya toh bas Kaafi hai
Download fast only for our Subscribers
DECODE DSA WITH PYTHON JAVA C++ SKILLS
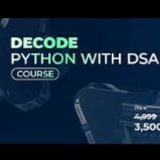
3,099 Subscribers
30 Photos
1 Videos
Last Updated 26.02.2025 01:31
Similar Channels

148,037 Subscribers

29,076 Subscribers

10,220 Subscribers
Decode DSA with Python, Java, and C++ Skills
In the realm of computer science, the concept of Data Structures and Algorithms (DSA) is fundamental to writing efficient and effective code. Mastery of DSA enables developers to not only enhance their programming skills but also to solve complex problems with relative ease. Data Structures, which are specialized formats for organizing, processing, and storing data, alongside Algorithms, which are step-by-step procedures for calculations, play a crucial role in various applications ranging from web development to artificial intelligence. In today's digital landscape, the ability to decode DSA using languages such as Python, Java, and C++ has become an invaluable skill for aspiring programmers and seasoned developers alike. This article aims to explore how these three popular programming languages can be utilized to understand and implement DSA concepts effectively, providing insights into their unique features and advantages, as well as addressing frequently asked questions around this topic.
What are Data Structures and why are they important?
Data Structures are a way of organizing and storing data in a computer so that it can be accessed and modified efficiently. They are crucial for managing large amounts of data and implementing algorithms that require quick data retrieval. By using appropriate data structures, developers can optimize performance in applications, leading to better user experiences and more scalable solutions.
The importance of data structures lies in their ability to affect the efficiency of algorithms. Different structures serve different purposes; for example, arrays allow fast access but are limited in size, whereas linked lists provide flexibility. Selecting the right data structure for a given problem can be the difference between a functional application and one that fails under load.
How do Python, Java, and C++ differ in implementing DSA?
Python's approach to DSA often emphasizes simplicity and readability, making it a popular choice among beginners. It has built-in data structures like lists, dictionaries, and sets, allowing developers to implement complex algorithms with less code. Python's extensive libraries, such as NumPy and pandas, also assist in handling data efficiently.
Java, on the other hand, is strongly typed and tends to be more verbose, which can lead to more rigorous data structure definitions. Java’s Collections Framework offers a rich set of classes for working with data structures like ArrayLists, HashMaps, and Trees, which helps in managing dynamic data efficiently. C++, being a lower-level language, provides greater control over memory management and performance, offering extensive Standard Template Library (STL) features that equip developers with powerful tools for data manipulation.
What are common algorithms used with Data Structures?
Common algorithms that frequently accompany data structures include sorting algorithms like QuickSort and MergeSort, search algorithms such as Binary Search, and graph algorithms like Dijkstra's or A* for pathfinding. These algorithms leverage data structures to operate efficiently based on the data organization, making their understanding pivotal for effective coding.
Moreover, dynamic programming algorithms like Fibonacci sequences or the Knapsack problem often utilize recursion and memoization techniques, highlighting the interplay between algorithms and data structures. Understanding these algorithms is essential as they are fundamental to solving many real-world problems in software development.
How can I improve my DSA skills using these programming languages?
Improving DSA skills can be accomplished through consistent practice and exposure to problem-solving. Online resources such as coding platforms (e.g., LeetCode, HackerRank, CodeSignal) provide numerous challenges that incorporate DSA concepts. By working through these problems in Python, Java, and C++, developers can gain familiarity with how different languages implement similar algorithms and data structures.
Additionally, reading books and tutorials focused on DSA can provide deeper theoretical knowledge. Participating in coding competitions or contributing to open source projects can further enhance practical skills, while communities and forums offer support and insights from fellow programmer enthusiasts.
What resources are recommended for learning DSA with Python, Java, and C++?
There are numerous resources available for learning DSA, including online courses, textbooks, and video lectures. Websites like Coursera, Udacity, and edX offer courses specifically focused on data structures and algorithms in various programming languages. Books such as 'Introduction to Algorithms' by Cormen and 'Data Structures and Algorithms Made Easy' by Narasimha Karumanchi are widely recommended.
YouTube also hosts countless tutorial series that break down complex topics in an accessible manner. Additionally, coding bootcamps and study groups can provide structured learning and collaboration opportunities, enhancing understanding through shared knowledge.
DECODE DSA WITH PYTHON JAVA C++ SKILLS Telegram Channel
DECODE DSA WITH PYTHON JAVA C++ SKILLS is a Telegram channel dedicated to helping individuals enhance their Data Structures and Algorithms (DSA) knowledge using Python, Java, and C++. Whether you are a beginner looking to grasp the basics or an experienced programmer aiming to deepen your understanding, this channel provides valuable resources and support. The channel offers a wide range of educational content, including tutorials, code snippets, practice exercises, and tips for optimizing DSA solutions using Python, Java, and C++. By joining this channel, you will have access to a community of like-minded individuals who are passionate about coding and eager to improve their DSA skills. Stay updated with the latest trends and techniques in DSA, ask questions, participate in discussions, and collaborate with fellow members to elevate your programming proficiency. Start your journey towards mastering DSA with Python, Java, and C++ today by joining DECODE DSA WITH PYTHON JAVA C++ SKILLS!